AI-derived data & techbio innovation: can AI-derived data provide evidence of plausibility of a therapeutic effect?
At the European Patent Office (EPO) a requirement for patentability is that the subject matter of the claims provides a technical effect.
In the field of life sciences this technical effect is often a therapeutic effect, with supporting evidence being required to establish that the claimed subject matter has a therapeutic effect.This can cause tension between pressures to file early, caused by the “first to file” approach to assessing novelty and the need to include supporting data, which may take some time to obtain. The EPO may accept post-published data as evidence of a technical effect, as discussed in our article “G 2/21: has anything changed?”.
However, the Enlarged Board of Appeal decided in G2/21 that “a patent applicant or proprietor may rely upon a technical effect for inventive step if the skilled person, having the common general knowledge in mind, and based on the application as originally filed, would consider said effect as being encompassed by the technical teaching and embodied by the same originally disclosed invention”. Therefore, it is important to include in the application as filed some evidence supporting an alleged therapeutic effect.
Traditionally, evidence of a therapeutic effect has required wet lab experimental data, but as we note in our article, “The rise of techbio and its IP needs: IP strategies for data-driven innovation”, in recent years data-driven solutions based on machine learning are increasingly being used to reduce the amount of wet lab research needed to identify novel compounds, targets or treatment regimes in the life science and biotech fields.
Related article
The rise of techbio and its IP needs: IP strategies for data-driven innovation
Read moreConsequently, if AI-derived evidence is considered enough to establish a therapeutic effect of a claimed invention, this might reduce the delay before a patent application can be filed, reducing risk of an invalidating prior art disclosure being made before filing.
It is likely that for the foreseeable future wet lab data will be considered more convincing than AI-derived data for establishing a therapeutic effect. Therefore, if wet lab data is available before filing, or it is feasible to obtain such wet lab data within acceptable time frames and costs, we would recommend including such wet lab data in a priority application. Further, in the absence of any official guidance on this point, it may be risky to rely solely on AI-derived data to support a technical effect, but it is possible that the EPO might (if not now, then in the future) accept some AI-derived data as supporting evidence for establishing a technical effect (possibly in combination with wet lab data).
As AI-derived data may be available earlier than corresponding wet lab data, one strategy could be to file an initial priority application early based on the AI data, with a view to obtaining further wet lab data within the twelve-month priority period, so that the wet lab data can be included in a subsequent filing claiming priority to the initial application. Post-published wet lab data could then also be used to further support the technical effect.
What AI-derived evidence could be used?
Let’s now consider what types of AI-derived information could be included in a patent application to support an argument that a therapeutic effect has been demonstrated in the initial application.
The EPO Technical Board of Appeal held in T1642/06 that for supporting a therapeutic effect, “it is not necessary for a therapeutic effect to have been demonstrated clinically [through clinical trials]”. Rather, it is sufficient that “the skilled person understands on the basis of generally accepted models that the results in the application directly and unambiguously reflect the claimed therapeutic applications”. This decision was in the context of the “generally accepted model” being an in vitro or animal model, but it seems reasonable to assume that the same could apply to data derived from AI models.
If AI-derived data is to be used to demonstrate a therapeutic effect in a priority application, we would therefore suggest including both:
- evidence derived from the AI model indicating that the claimed subject-matter is predicted by the model to have the stated therapeutic effect; and
- evidence for why the AI model should be regarded as a “generally accepted model” capable of making good predictions.
For point 1, if the model is a scoring model which assigns a quantitative score to each candidate compound or treatment, data could be provided showing the score assigned to the claimed invention in comparison to other inferior candidates. For a classification model, the application could simply identify that the model assigned the “good candidate” class to the claimed invention (differentiating from other candidates assigned the “bad candidate” class). This should be fairly straightforward to establish.
However, providing good evidence of a “generally accepted model” for point 2 may take more effort. First, we recommend describing in detail how the model was trained (see, for example, our guide Computer implemented inventions at the EPO: patent application tips). We suggest describing the type of model used, how the training data set was obtained, and the steps taken to train the model using the data set and verify model prediction performance.
During the training some model performance metrics may be measured for assessing the model’s predictive capability. For example, for regression models a metric such as root mean squared error can be used to express the distance between the model’s predictions and the ground truth of the corresponding training examples. For classification models, which classify each input into one of a set of discrete classes, a confusion matrix could be generated to express, for each combination of a ground truth class expressing the true nature of a training example and a predicted class assigned by the model to that training example, the fraction of instances of a training example with that ground truth class for which the model predicted that predicted class. Alternatively, a quantitative measure may express the ratio of total predictions which are considered “good” (for example, taking into account true positive, true negative, false positive and/or false negative predictions). It may be advisable to include in the patent application some performance metrics as evidence of the model’s predictive performance.
However, such metrics may not be enough on their own to establish that the AI data is prepared using an accepted model. A model trained based on a biased training data set might have good performance metrics, but nevertheless make predictions that fare poorly in the real world. For instance, a model may have learned to predict the occurrence of noise unrelated to the therapeutic effect, or could become over-fitted to particular quirks of the training data set used, so may be less useful at making predictions for new examples not in the training data set.
Therefore, in addition to discussing model performance metrics, we also recommend including in the initial patent application a description of steps taken to reduce risk of data set bias or over-fitting. For example, the patent application could discuss how the training data set was obtained from multiple sources or based on a wide variety of test subjects. It may be useful to describe cross-validation of the model with different training runs being performed using different subsets of training examples taken from a larger data set, with some quantitative analysis of whether the model’s predictive performance remains consistent across different training runs (a sign of a model more likely to provide useful predictions when applied to new examples not in the original training set). The patent application could also include analysis of model complexity: if a model can give acceptable performance with fewer variables being trained, it may be less prone to over-fitting than a model with a large number of variables being trained.
To have the best possible chance of AI-derived data being considered to demonstrate a therapeutic effect, any information available from the inventors about how they established whether the model can make reliable predictions should be included in the initial application. However, this will need to be balanced with whether the applicant/inventors are happy for such information about their machine learning model to be published in a patent application.
Summary
While wet lab experimental evidence is likely to be more convincing than AI-derived data for demonstrating a therapeutic effect, if only AI-derived evidence is available at the point you wish to file the application we would recommend considering whether such data may be adequate. If this approach is followed consideration should to be given on how it can be established that the AI model is an accepted model. Currently, we consider that the AI-derived data would need to be followed with wet lab data either within the priority year or beyond.
Related articles
- G 2/21: has anything changed?: dycip.com/g221changes
- The rise of techbio and its IP needs: IP strategies for data-driven innovation: dycip.com/techbio-ip-datadriven-innovation
- T 1642/06, EPO Technical Board of Appeal, 23 August 2007: dycip.com/t-1642-06
- Computer implemented inventions at the EPO: patent application tips: www.dyoung.com/cii-patent-tips
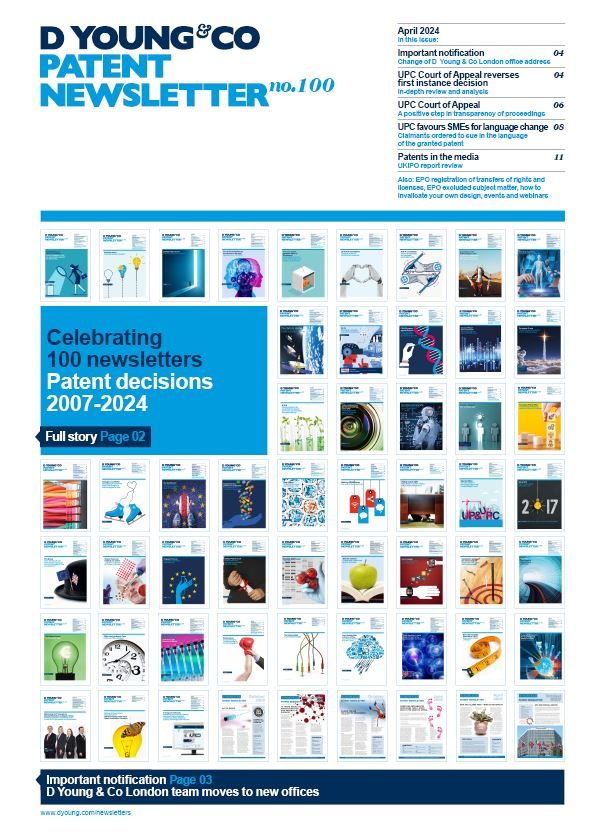