AI in drug discovery - technical and IP challenges
Artificial intelligence (AI) and machine learning (ML) are not new concepts – they have been the subject of academic investigation for decades. However, real-world applications have had to wait longer for the availability of the computing power and rich data sets necessary to successfully implement such approaches.
In recent years there has been an explosion in the number of start-ups offering AI or ML based solutions that promise to improve the efficiency of the drug discovery process. There is undoubtedly much value to be added here. While the causes are the subject of much debate, it is clear that the time and financial costs of bringing a new drug to market continue to increase. Further, the development of new technologies such as high-throughput sequencing and “omics” that provide large volumes of rich data, together with the “publish or perish” culture of academic research mean that those working in drug discovery are faced with an ever growing mountain of complex data to navigate in their search for a lead.
We recently attended “Accelerating Drug Discovery through Digital and AI Innovations” organised by the UK Bioindustry Association and “Artificial Intelligence in Chemistry” organised by the Royal Society of Chemistry to learn more about this exciting area and to understand the challenges as well as value offered by using AI and ML in drug discovery.
Finding hidden connections
Machine learning algorithms can be particularly good at finding hidden connections within large, complex data sets that may go undiscovered by human eyes. Several start-ups are offering platforms that leverage data from multiple sources such as public and proprietary databases, academic and patent literature, patient and clinical data as well as expert input and curation. Such data can then be analysed using approaches such as natural language processing (NLP) to identify and catalogue entities and then search for “connections”, such as biological pathways with potential for pharmacological intervention.
Other data sets being successfully mined are large databases of nucleic and amino acid sequences. The RSC event included a fascinating presentation by Alexander Pritzel of DeepMind discussing their highly publicised AlphaFold2 model which uses sequence information and known protein structures to predict protein structures with staggering results. With experimental routes to determining protein structures such as X-ray crystallography and cryo-EM being so time consuming and challenging, such AI approaches herald a step-change in the life sciences.
AI can also be used to identify new compounds or identify existing drugs that might be repurposed or further refined. Moreover, compounds might also be screened to predict how they might perform in the clinic, to concentrate on compounds with the most promising safety profiles, for example. Finally, AI or ML approaches are also being developed to provide new or improved synthesis methods.
The search for quality over quantity in drug discovery
Artificial intelligence and machine learning approaches offer the potential to examine a large number of aspects of the drug discovery pipeline at an early stage before compounds need to be taken into the laboratory. This may allow a smaller number of more promising compounds to be taken forward for development, leading to a focus on quality over quantity. In turn, the hope is that while more compounds may be expected to fail earlier, the pipeline should become more efficient as more resources can be dedicated to the most promising leads.
AstraZeneca, for example, has implemented its “5R framework” to improve R&D productivity. The framework focuses on five key determinants: the right target, right tissue, right safety, right patient, and right commercial potential, which in a number of aspects draw heavily on the use of technologies such as AI and ML to focus on the quality of hits entering the laboratory pipeline. Put simply, it is too expensive to turn the handle ever faster, and AstraZeneca has moved to evaluating targets more extensively and earlier on, so that fewer, but higher quality, projects are taken forward. Since the implementation of the 5R framework, AstraZeneca reports that success rates from candidate drug nomination to phase III completion have improved from 4% in 2005–2010 to 19% in 2012–2016 [see note 1 at the end of this article].
Technical challenges
Artificial intelligence and machine learning is in many respects already mature. In applying these approaches to drug discovery, however, a significant challenge is the quality of data sets. While some published studies may be erroneous or not reproducible, there are also more mundane but still significant challenges around the standardisation of identifiers and data formats.
Patrick Walters of Relay Therapeutics gave a fascinating talk in which he discussed some of the challenges associated with representing molecules in AI models. For example, in light of the already significant complexity of these approaches, it is often necessary to represent compounds in two dimensions, but of course molecules are three dimensional, often with multiple conformers, and so this simplification can cause difficulties. Another challenge is how to remove nonsensical chemical structures from the model, whilst still identifying interesting new compounds for development.
Another challenge is that many data sets are proprietary. While it is widely agreed that collaboration is important to improve the application of AI and ML in drug discovery, complex issues of data sharing and ownership will need to be resolved. Finally, many current technologies have been validated retrospectively, that is, after a discovery programme has already started running, and the field currently lacks good examples of prospective leads from the outset.
Intellectual property challenges
While legal issues raised by the concept of an “AI inventor” have recently been the subject of debate, and are of great interest to legal practitioners, in drug discovery at least the focus is on when and how to protect AI and ML technologies as tools for automation and improvement of the drug discovery process.
Companies developing or using AI and ML-based technologies for drug discovery potentially have two distinct groups of property that they may wish to protect. Molecules derived from such programmes can of course be protected by patents subject to the applicable requirements of patentability. However, companies may also wish to protect their algorithms and associated technology. Two options that might be considered are patents and trade secrets.
At the European Patent Office, artificial intelligence and machine learning appear at first instance to fall within “mathematical methods” such that they would be excluded from patentability. Indeed, an invention relating solely to a fundamental advance in AI/ML intrinsically would not be patentable. However, AI and ML methods that provide a technical solution to a technical problem are generally considered patentable. Careful thought must therefore be given when drafting an application to ensure that the AI or ML method is suitably tied into the solution provided by the invention, for example the identification of a new drug. As this is a developing area, we would expect the EPO guidance to evolve further as new judgments from the Board of Appeal become available.
In some cases, companies working in the AI or ML drug discovery space may choose to rely on trade secret or know-how provisions to protect their methods and reserve patent applications for the hits discovered by their use. Indeed, a trade-off of patenting is that your invention is put into the public domain once the patent application is published. Keeping a technology as a trade secret may indeed be a viable option if it is hard to reverse engineer and can be kept confidential for a long period of time. However, there is of course no protection against a competitor independently developing the same technology, which may be a particular risk in a fast moving field.
Clients working in this area should talk to an IP professional early on to discuss how best to protect their innovation. A cross-disciplinary approach involving professionals working in both the pharma and information technology sectors is likely to be most effective. For further information please contact Catherine Keetch or your usual D Young and Co representative.
Note
- Morgan, P., Brown, D., Lennard, S. et al. Impact of a five-dimensional framework on R&D productivity at AstraZeneca. Nat Rev Drug Discov 17, 167–181 (2018). https://doi.org/10.1038/nrd.2017.244
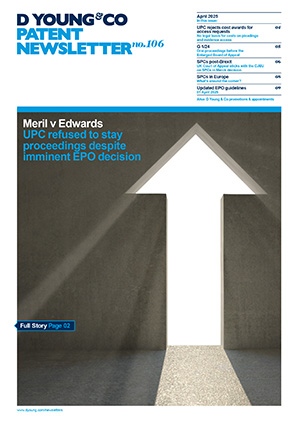
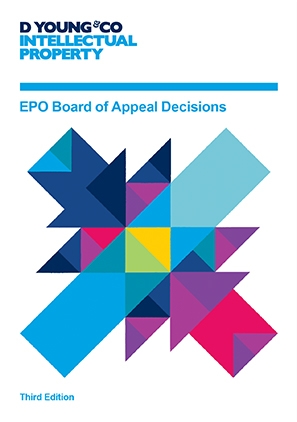